Research Newsletter: The Imperative of Cloud & IT Auditing for AI/ML Development
As the adoption of Artificial Intelligence (AI) and Machine Learning (ML) soars across industries, ensuring the security, transparency, and reliability of these technologies is paramount. One cannot overlook the pivotal role of cloud and IT auditing in achieving this goal. This newsletter provides a comprehensive case study into why these audits are indispensable for AI and ML model development.
Fellow Member: Joshua Leo
12/2/20222 min read
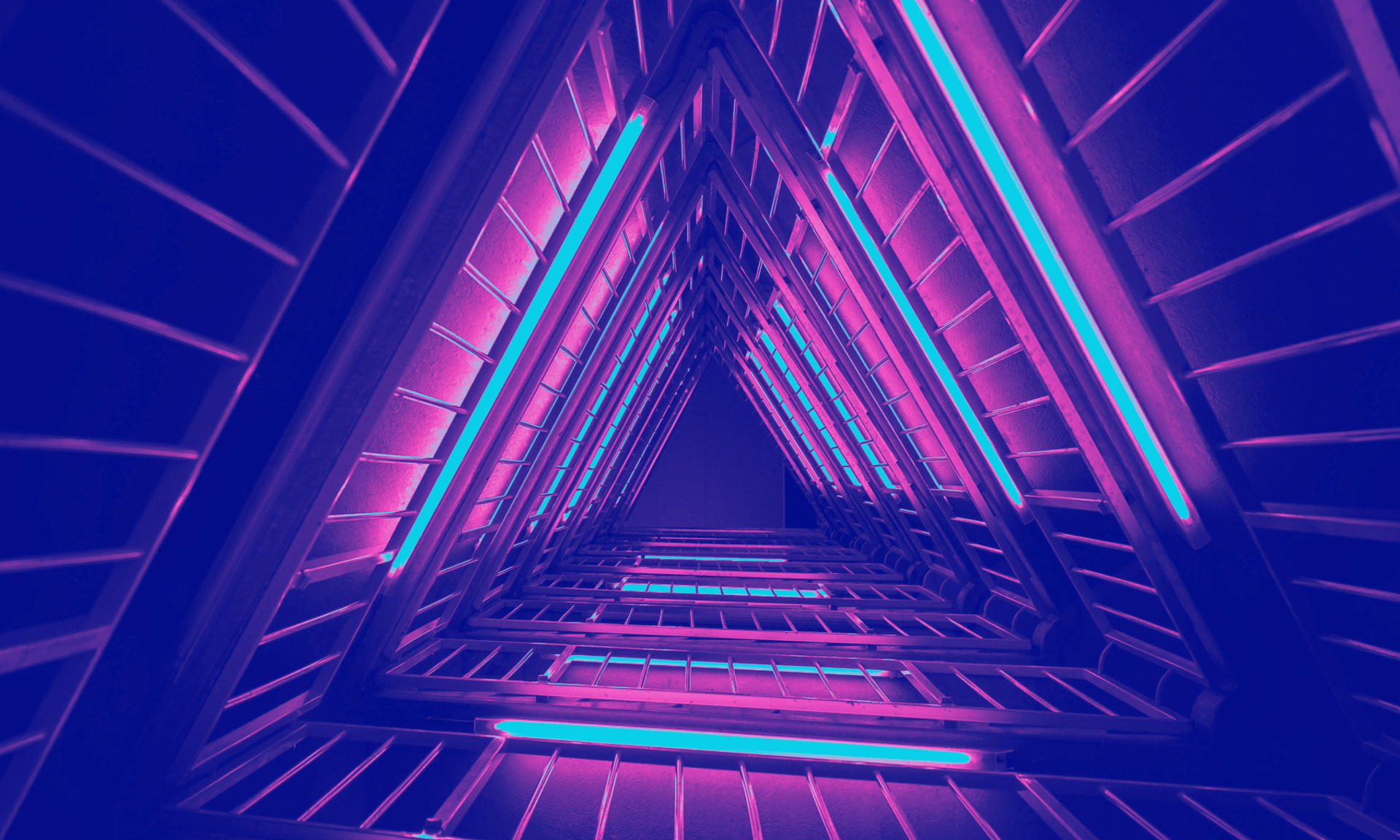
1. The Explosion of Cloud-based AI/ML Services
Modern AI/ML frameworks largely rely on cloud infrastructures for data storage, processing, and model training. Studies like those from [Gartner (2019)](https://www.gartner.com/en) indicated a massive spike in AI/ML services hosted on cloud platforms. The ease of scalability, cost-effectiveness, and accessibility makes the cloud a preferred choice. However, with growth come challenges, especially concerning security and compliance.
2. The Risks: Why We Can't Overlook Cloud & IT Auditing
2.1 Data Breaches and Loss
AI/ML models are trained on vast amounts of data. This data, if not adequately secured, is susceptible to breaches. A study from [Ponemon Institute (2020)](https://www.ponemon.org/) highlighted the escalating costs and frequency of data breaches in cloud environments.
2.2 Model Integrity
A compromised cloud infrastructure can lead to manipulated models. Adversarial attacks, where threat actors introduce malicious data to alter model behavior, are becoming commonplace, as noted by [Biggio et al. (2018)](https://arxiv.org/abs/1810.00069).
2.3 Compliance and Regulatory Risks
Organizations must adhere to data protection laws like GDPR and CCPA. Non-compliance not only poses reputational risks but also entails hefty fines.
3. Benefits of Cloud & IT Auditing in AI/ML Development
3.1 Enhanced Security
Auditing identifies vulnerabilities in the infrastructure, thereby enabling organizations to bolster their security. An effective audit can prevent unauthorized access, data leakage, and maintain the integrity of AI/ML models.
3.2 Compliance Assurance
Regular audits ensure that AI/ML services comply with international standards and regulations. They assist organizations in keeping up with the evolving regulatory landscape.
3.3 Trust and Reliability
Transparency achieved through rigorous auditing fosters trust among stakeholders, including customers, partners, and regulators. It assures them of the reliability of AI/ML solutions.
4. Case in Point: Cloud Misconfiguration in a Leading AI Firm
In 2021, a renowned AI-driven company experienced a significant data breach due to cloud misconfigurations. The breach exposed millions of sensitive records. Investigations revealed that simple security measures, if implemented, could have averted the mishap. An IT audit would have detected these misconfigurations. The incident underlines the significance of regular audits in preempting such vulnerabilities.
5. Moving Forward: Best Practices
5.1 Regular Audits
Conduct cloud and IT audits at regular intervals. Using tools like AWS's Guard Duty or Azure's Security Center can automate vulnerability detection.
5.2 Collaborate with Experts
Engage with third-party auditing firms specializing in AI/ML and cloud environments to ensure unbiased and thorough evaluation.
5.3 Continuous Training
Regularly train IT teams on the best security practices and emerging threats in the AI/ML domain.
Conclusion
The symbiosis of AI/ML with cloud platforms promises unprecedented advancements. However, with mounting complexities, the need for robust cloud and IT auditing has never been more pressing. By embracing proactive and systematic auditing, organizations can navigate the challenges, ensuring the secure, transparent, and efficient deployment of AI/ML solutions.
References
1. Gartner (2019). "Cloud Services Market Trends".
2. Ponemon Institute (2020). "Cost of a Data Breach Report".
3. Biggio, B., & Roli, F. (2018). "Wild Patterns: Ten Years After the Rise of Adversarial Machine Learning". arXiv preprint arXiv:1810.00069.
Contact us
For inquiries, collaborations, or assistance, reach out using our contact form. We're here to assist and collaborate with you.
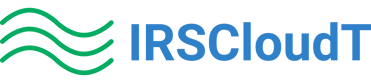
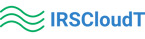
Disclaimer Notice
Keep in Touch
contact [AT] irsct [DOT] org
415 High Street
Stratford, London E15 4QZ
United Kingdom
Important Links
Copyright ©2023 by International Research Scholars for Cloud Technology (IRSCT) – All rights reserved. Your use of this site and the provisions of information are guided by our disclaimer, terms and conditions, and privacy policy. By accessing this site, you confirm your understanding and agreement with these terms. The contents of the research materials and journals reflect the views of their respective authors, with no implied endorsement or guarantee from IRSCT. All logos, trademarks, and names on this site are the sole properties of their respective owners. IRSCT holds a firm stance against plagiarism. Any detected instance will render the involved authors solely accountable, potentially facing legal repercussions. Please note that all information, activities, materials, and services, including those of this website, are subject to modification at any given time without prior notification.