Generative AI and Cloud Technology: Converging Frontiers of Innovation
Generative AI, a subset of artificial intelligence, specializes in producing new data mimicking existing data patterns. With the exponential increase in the volume of data and computational demands, cloud technology provides an optimal platform for the development and deployment of Generative AI models. This article elucidates the symbiosis between Generative AI and Cloud Technology, highlighting advantages, challenges, and prospective avenues.
Member: Dave Pertoci
8/18/20233 min read
Generative AI and Cloud Technology: Converging Frontiers of Innovation
1. Introduction
The realm of Generative Artificial Intelligence (AI) encompasses algorithms and models designed to generate complex patterns and structures, such as images, sounds, and texts. Notably, Generative Adversarial Networks (GANs) and Variational Autoencoders (VAEs) stand as iconic models in this sphere. However, the computational demands of these models are massive. Herein lies the role of cloud technology, providing scalable infrastructure and democratizing access to high-performance computing resources.
2. Generative AI: A Brief Overview
Generative AI's primary goal is to understand data distributions and produce new instances from these understood distributions. This is often achieved by:
2.1. Generative Adversarial Networks (GANs): Comprising two neural networks - the Generator and the Discriminator - they work in tandem, with the former generating data and the latter distinguishing generated data from real data.
2.2. Variational Autoencoders (VAEs): These are probabilistic models that reconstruct input data, effectively capturing the data distribution in a latent space, facilitating the generation of new instances.
3. The Cloud Paradigm in AI Research
The cloud brings forth several advantages to the AI research community:
3.1. Scalability: Platforms like AWS, Google Cloud, and Microsoft Azure offer elastic compute resources, enabling researchers to scale up or down based on demand, which is essential for training large generative models.
3.2. Collaboration: Cloud platforms provide a unified environment where researchers across the globe can collaboratively design, train, and deploy models.
3.3. Cost-Efficiency: Instead of investing in expensive local hardware, researchers can leverage pay-as-you-go models, which can be more cost-effective, especially for sporadic or short-term projects.
4. Confluence of Generative AI and Cloud: Synergies and Advantages
The convergence of Generative AI and cloud technology heralds a plethora of benefits:
4.1. Enhanced Model Training: With cloud's virtually unlimited computational resources, training complex models like GANs, which require substantial GPU/CPU capacities, becomes feasible.
4.2. Data Storage Solutions: Generative models necessitate extensive datasets. Cloud platforms offer robust and scalable storage solutions such as AWS S3 or Google Cloud Storage, ensuring data availability and integrity.
4.3. Democratizing AI: Cloud technology has democratized access to generative AI by offering Machine Learning as a Service (MLaaS) solutions. For instance, platforms like Azure Machine Learning enable users to develop, train, and deploy AI models without in-depth knowledge of the underlying algorithms.
5. Challenges and Limitations
While the convergence brings numerous advantages, some challenges need addressing:
5.1. Latency Issues: Real-time applications using generative models can experience latency, especially if data retrieval and processing happen across distributed cloud nodes.
5.2. Security Concerns: Storing data on cloud platforms can expose vulnerabilities, risking data breaches. Ensuring data encryption and compliance becomes paramount.
5.3. Cost Overheads: Despite being cost-effective, unrestricted use of cloud resources, especially for prolonged model training, can lead to unexpected costs.
6. Future Directions
The intersection of Generative AI and cloud technology promises several intriguing future avenues:
6.1. Generative Cloud Platforms: Dedicated cloud platforms optimized for generative AI tasks, offering specialized hardware accelerators and reduced training times.
6.2. Real-time Generative Applications: Leveraging edge computing in tandem with cloud resources to facilitate real-time generative applications, especially in AR, VR, and multimedia.
6.3. Enhanced Privacy Protocols: With generative models being utilized in sensitive applications, devising cloud protocols ensuring data privacy and model integrity is crucial.
7. Conclusion
The fusion of Generative AI and cloud technology is shaping a transformative frontier in the AI landscape. As we navigate the challenges, the potential for innovation and impact is profound. With continued collaboration between AI researchers and cloud providers, the promise of this synergy will undoubtedly unfold in unprecedented ways.
Contact us
For inquiries, collaborations, or assistance, reach out using our contact form. We're here to assist and collaborate with you.
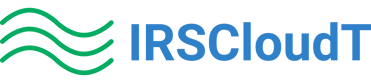
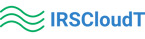
Disclaimer Notice
Keep in Touch
contact [AT] irsct [DOT] org
415 High Street
Stratford, London E15 4QZ
United Kingdom
Important Links
Copyright ©2023 by International Research Scholars for Cloud Technology (IRSCT) – All rights reserved. Your use of this site and the provisions of information are guided by our disclaimer, terms and conditions, and privacy policy. By accessing this site, you confirm your understanding and agreement with these terms. The contents of the research materials and journals reflect the views of their respective authors, with no implied endorsement or guarantee from IRSCT. All logos, trademarks, and names on this site are the sole properties of their respective owners. IRSCT holds a firm stance against plagiarism. Any detected instance will render the involved authors solely accountable, potentially facing legal repercussions. Please note that all information, activities, materials, and services, including those of this website, are subject to modification at any given time without prior notification.