Emerging AI Companies and Cloud Services: Harnessing the Future
Emerging AI companies are increasingly leveraging cloud services to drive innovation. This article explores the synergy between AI startups and cloud platforms, highlighting key features such as scalable infrastructure, GPU acceleration, and MLaaS. Real-world applications and challenges, including cost management and vendor lock-in, are also discussed.
Member: Mr. Ziang Shyo
8/29/20233 min read
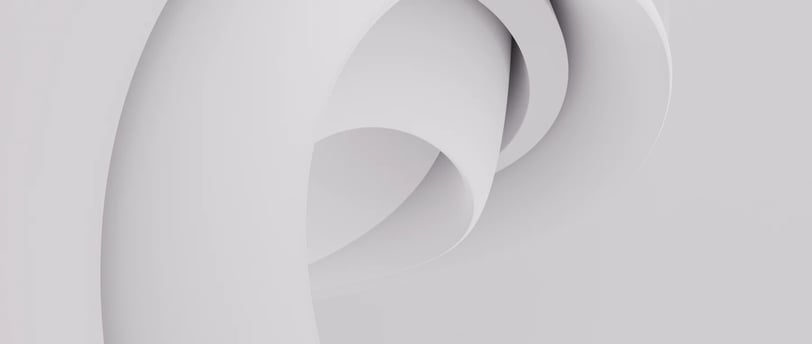
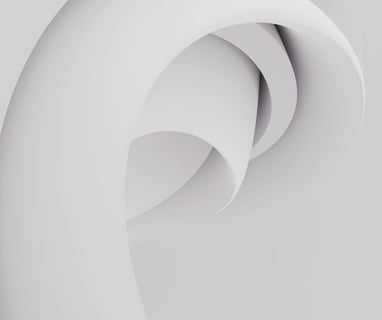
Introduction
Artificial Intelligence (AI) is reshaping industries, driving innovation, and introducing efficiencies previously deemed unattainable. At the core of this transformation are emerging AI companies, agile entities pushing the boundaries of what’s possible. Aiding them in this pursuit is cloud technology, providing scalability, flexibility, and a host of features that make AI deployment more accessible and impactful. This article delves into the synergy between AI startups and cloud services, focusing on the key cloud features they utilize.
1. Cloud Services: An AI Catalyst
For emerging AI companies, cloud platforms offer an unparalleled advantage. With vast computational power, storage, and specialized tools at their fingertips, startups can focus on algorithmic innovation rather than infrastructure management.
2. Key Cloud Features Leveraged by AI Startups
2.1. Scalable Infrastructure: Cloud platforms like AWS, Google Cloud, and Azure allow companies to scale their computational resources dynamically. This elasticity is crucial for AI tasks which can vary in demand, from data preprocessing to intensive model training sessions.
2.2. GPU Acceleration: Training deep learning models require significant computational power, often provided by Graphics Processing Units (GPUs). Cloud platforms offer GPU instances, such as AWS’s EC2 P3 or Google’s Compute Engine with NVIDIA GPUs, which can significantly reduce training times.
2.3. Pre-trained Models and ML Services: Platforms offer Machine Learning as a Service (MLaaS), where companies can leverage pre-trained models for tasks like image recognition (e.g., Google Vision AI) or natural language processing (e.g., Amazon Comprehend). This allows startups to bootstrap AI functionalities without starting from scratch.
2.4. Data Lakes and Storage: Storing, retrieving, and processing vast amounts of data is pivotal for AI applications. Services like AWS Lake Formation or Azure Data Lake allow firms to set up scalable and secure data lakes efficiently.
2.5. Development and Collaboration Tools: Cloud platforms provide a suite of tools that facilitate AI development, such as TensorFlow on Google Cloud or SageMaker on AWS. These tools, combined with collaborative platforms, enable decentralized teams to work together seamlessly.
2.6. Edge AI Services: With the rise of IoT devices and the need for on-site AI computations, edge AI has gained prominence. Cloud platforms support this through services like AWS Greengrass or Azure IoT Edge, allowing AI models to run on edge devices while maintaining cloud connectivity for management.
2.7. Security and Compliance: Data privacy and model integrity are paramount. Cloud providers offer robust security features, including data encryption, secure access protocols, and compliance certifications, ensuring AI operations meet industry standards and regulations.
3. Real-world Applications: AI Startups in Action
3.1. Healthcare Diagnostics: AI startups, like PathAI, are leveraging cloud's computational prowess to process medical images and provide rapid diagnostic insights. They utilize GPU acceleration for real-time processing and data lakes to handle vast patient datasets.
3.2. Financial Analytics: Fintech AI firms, such as Upstart, use cloud platforms for credit decision algorithms, leveraging MLaaS for risk modeling and assessment. The scalability feature ensures they can handle spikes during high transaction periods.
3.3. AgriTech Innovations: Startups like AgroStar harness cloud services to process satellite imagery and provide insights to farmers on crop health, leveraging the vast storage capabilities and GPU instances for real-time analytics.
4. Challenges and Considerations
While the synergy between AI startups and cloud services offers immense advantages, there are challenges:
4.1. Cost Management: The pay-as-you-go model can lead to unexpected costs if not carefully managed, especially with extensive data processing or prolonged training sessions.
4.2. Vendor Lock-in: Relying heavily on a single cloud provider’s tools and services might make migration difficult if a company decides to switch platforms.
4.3. Data Transfer Bottlenecks: Moving vast amounts of data to and from the cloud can be time-consuming, potentially slowing down AI workflows.
5. Conclusion
Emerging AI companies are not just riding the wave of technological advancement – they are at the forefront, steering its direction. Cloud services, with their vast array of features tailored for AI, act as the wind beneath their wings. As the landscape evolves, this symbiotic relationship between AI startups and cloud platforms will undoubtedly deepen, unlocking new horizons of innovation.
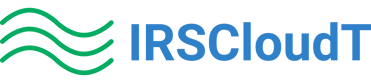
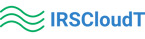
Disclaimer Notice
Keep in Touch
contact [AT] irsct [DOT] org
415 High Street
Stratford, London E15 4QZ
United Kingdom
Important Links
Copyright ©2023 by International Research Scholars for Cloud Technology (IRSCT) – All rights reserved. Your use of this site and the provisions of information are guided by our disclaimer, terms and conditions, and privacy policy. By accessing this site, you confirm your understanding and agreement with these terms. The contents of the research materials and journals reflect the views of their respective authors, with no implied endorsement or guarantee from IRSCT. All logos, trademarks, and names on this site are the sole properties of their respective owners. IRSCT holds a firm stance against plagiarism. Any detected instance will render the involved authors solely accountable, potentially facing legal repercussions. Please note that all information, activities, materials, and services, including those of this website, are subject to modification at any given time without prior notification.